To better understand some of the artworks that I am working with I also need some more technical understanding of Machine learning with images, AI and neural networks. Below I collected some of my readings and notes from last week.
Machine vision and AI
Computer science: The learning machines
Nicola Jones
08 January 2014
https://www.nature.com/news/computer-science-the-learning-machines-1.14481
– Google Brain case (deep neural network)
– Deep learning
– Short review of computer vision developments (rule based to neural networks)
– ImageNet competition
– crowd-sourced online game called EyeWire (https://eyewire.org/) to map neurons in the retina
– “Deep learning happens to have the property that if you feed it more data it gets better and better,”
– Modeling the brain might not be the best solution, there are other attempts like “reason on inputted facts” (IBM’s Watson).
– Probably deep learning is going to be combined with other ideas.
Building High-level Features
Using Large Scale Unsupervised Learning
Quoc V. Le, Marc’Aurelio Ranzato, Rajat Monga, Matthieu Devin, Kai Chen, Greg S. Corrado, Je Dean, Andrew Y. Ng
12 Jul 2012
https://arxiv.org/pdf/1112.6209.pdf
– A paper on improving face recognition (also cats and body parts)
– How deep learning is a methodology to build features from “unlabeled data” (self-taught learning not supervised or rewarded)
– Core components in deep networks: dataset, model and computational resources.
– Uses sampling frames from 10 million Youtube Videos
Dermatologist-level classification of skin cancer with deep neural networks
Andre Esteva, Brett Kuprel, Roberto A. Novoa, Justin Ko, Susan M. Swetter, Helen M. Blau & Sebastian Thrun
02 February 2017 (A Corrigendum to this article was published on 28 June 2017)
https://www.nature.com/articles/nature21056
– Deep convolutional neural network and a dataset of 129450 clinical images used to train machines to recognized skin cancer.
– Future possible application to make low-cost diagnosis with smartphone.
Can we open the black box of AI?
Davide Castelvecchi
05 October 2016
https://www.nature.com/news/can-we-open-the-black-box-of-ai-1.20731#sheep
– with historical perspective how neural networks developed
– networks are as much a black box as the brain
– neural networks and brains do not store information in blocks of digital memory, they diffuse the information which makes it hard to understand.
– “some researchers believe, computers equipped with deep learning may even display imagination and creativity”
– Deep Dream how networks produce images
– we are as far from understanding these networks as we are from understanding the brain
– therefore used with caution (cancer example)
– the network has understood something but we have not
– to understand how the network was learning they ran the training in reverse -> same approach with Deep Dream (artworks Tyka?). Deep Dream made available online.
– “fooling” problem causes problems – hackers could fool cars drive into billboards thinking they were streets etc.
– “You use your brain all the time; you trust your brain all the time; and you have no idea how your brain works.”
BIAS and AI
AI can be sexist and racist — it’s time to make it fair
James Zou & Londa Schiebinger
18 July 2018
https://www.nature.com/articles/d41586-018-05707-8#ref-CR10
– Bias is not unique, but increased use of AI makes it important to address
– Skwed data:images by scraping Google images or Google News, with specific terms, annotated by graduate students or Amazons Mechanical Turk leads to data that is encode gender, ethnic and cultural biases.
– ImageNet that is most used in computer vision research has 45% of its material from USA (home to only 4%), China and India with much bigger populations contributes just a fraction of the data.
– Examples: Skin color in cancer research, western bride vs. Indian bride, misclassify gender of darker-skinned women.
– Suggested “fixes”: Diverse data, standardized metadata as a component of an peer-review-process, retraining, in crowd sourced labeling basic information about the participants should be included and information about their training. Another algorithm testing the bias of the learning algorithm (AI audit), designers of algorithms prioritizing to avoid biases.
– ImageNet 14 million labeled images USA 45.4%, Great Britain 7,6% Italy 6.2%, Canada 3% Other 37.8%
– Who should decide which notions of fairness to prioritize?
– Human-Centered Ai initiative in Standford University CA (social context of AI)
Bias detectives: the researchers striving to make algorithms fair
Rachel Courtland
https://www.nature.com/articles/d41586-018-05469-3
20 June 2018
– About predictive policing and AI
– AI Now Institute in New York University (social implications of AI)
– A task force in NYC investigating how to publicly share information about algorithms to examine how bias they are.
– GDPR in Europe is expected to promote algorithmic accountability.
– Enhancing exciting inequality e.g. use of police records in the states. The algorithm is not bias, but the data and how it was collected in the past.
– Questions of how define fair
– Examples of Auditing AI from outside (mock passengers, dummy cv’s etc.)
– Researchers asking if we are trying to solve the right problems (predicting who is appearing in court vs. how to support people to appear in court)
Investigating the Impact of Gender on Rank in Resume
Search Engines
Le Chen, Ruijun Ma, Anikó Hannák, Christo Wilson
https://dl.acm.org/citation.cfm?doid=3173574.3174225
– Overview on studies on algorithmic biases (AI audits).
– Investigating gender inequality in Job Search Engines
– Concludes that there is unfairness but the audit can not isolate the hidden features that may be causing it.
– A lot of limitations in these types of AI audits
Reform predictive policing
Aaron Shapiro
25 January 2017
https://www.nature.com/news/reform-predictive-policing-1.21338
– Overview of how predictive policing is currently used
– Overview of tech used in predictive policing and what kind of data is used:
“The data include records of public reports of crime and requests for police assistance, as well as weather patterns and Moon phases, geographical features such as bars or transport hubs, and schedules of major events or school cycles.”
– Concerns: no agreement on should predictive systems prevent crime or catch criminals, racial bias in crime data and bias on reported crime data.
There is a blind spot in AI research
https://www.nature.com/news/there-is-a-blind-spot-in-ai-research-1.20805
Kate Crawford & Ryan Calo
13 October 2016
– no agreed methods to assess how AI effect on human populations (in social, cultural and political settings)
– The rapid evolving of AI has also increased demand for so called “social systems analyses of AI”.
– No consensus on what counts as AI
– Tools to avoid bias: compliance, ‘values in design’ and thought experiments
– Examples of images and algorithms: Google mislabeling African americans with gorillas and Facebook censoring Pulitzer-prizewinning photograph with a naked girl fleeing the napalm attack in Vietnam.
– Suggests a social-systems analysis that examines AI impact on several states: conception, design, deployment and regulation
The Dataset Nutrition Label: A Framework To Drive Higher Data Quality Standards
Sarah Holland, Ahmed Hosny, Sarah Newman, Joshua Joseph and Kasia Chmielinski
Draft from May 2018
https://arxiv.org/ftp/arxiv/papers/1805/1805.03677.pdf
Prototype: https://ahmedhosny.github.io/datanutrition/
– Survey on best practices of ensuring quality of data to train AI
– Survey shows no best practices, self learned
– “Dataset Nutrition Label” inspired by labeling food, “Privacy Nutrition Label” from World Wide Web Consortium improved legibility of privacy policies.
– Ranking Facts labels algorithms
– “Datasheets for Datasets,” including dataset provenance, key characteristics, relevant regulations and test results, potential bias, strengths and weaknesses of the dataset, API or model and suggested use.
– Data Statements for NLP (natural language processing)
– “Dataset Nutrition Label” should improve data selection and therefore result in better models.
Datasheets for Datasets
Timnit Gebru, Jamie Morgenstern, Briana Vecchione, Jennifer Wortman Vaughan, Hanna Wallach, Hal Daumé III, Kate Crawford
9th Jul 2018
https://arxiv.org/pdf/1803.09010.pdf
– Another initiative to standardize datasets used in machine learning based on practices in electronics industry.
Anticipating artificial intelligence
Editorial
26 April 2016
https://www.nature.com/news/anticipating-artificial-intelligence-1.19825
– Researchers concerned about AI (Luddite Award)
– Not that machines and robots outperform humans, but:
– the surveillance and loss of privacy can be amplified
– drones making lethal decisions by themselves
– smart cities, infrastructures and industry becoming overdependent on AI
– mass extensions in jobs, leading to new types of jobs and societal change (permanent unemployment increases inequality)
– Could lead to wealth being even more concentrated if gains from productivity is not shared.
Who is working with AI/Machine vision
AI talent grab sparks excitement and concern
Elizabeth Gibney
26 April 2016
https://www.nature.com/news/ai-talent-grab-sparks-excitement-and-concern-1.19821
– Academia vs. Industry
– From where (which Universities) talent is moving to industry
Images
Can we open the black box of AI?
Source: https://www.nature.com/news/can-we-open-the-black-box-of-ai-1.20731
‘Do AIs dream of electric sheep?’
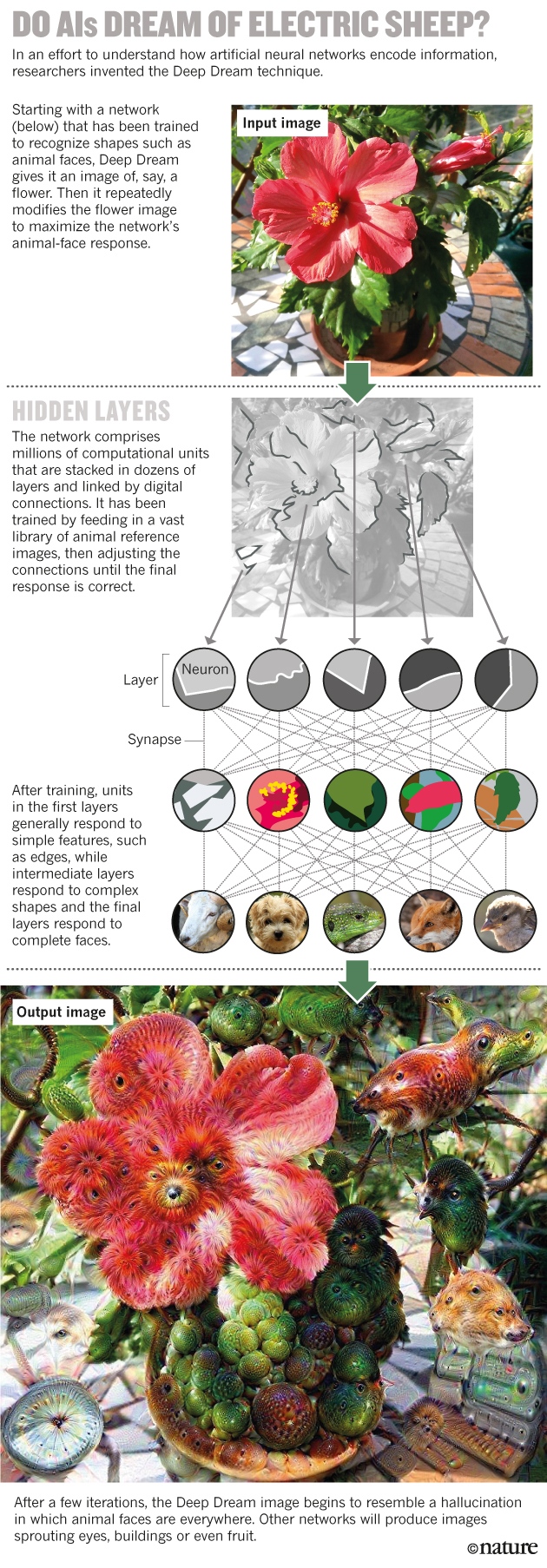
A world where everyone has a robot: why 2040 could blow your mind
Source: https://www.nature.com/news/a-world-where-everyone-has-a-robot-why-2040-could-blow-your-mind-1.19431
Onwards & Upwards

Predictions
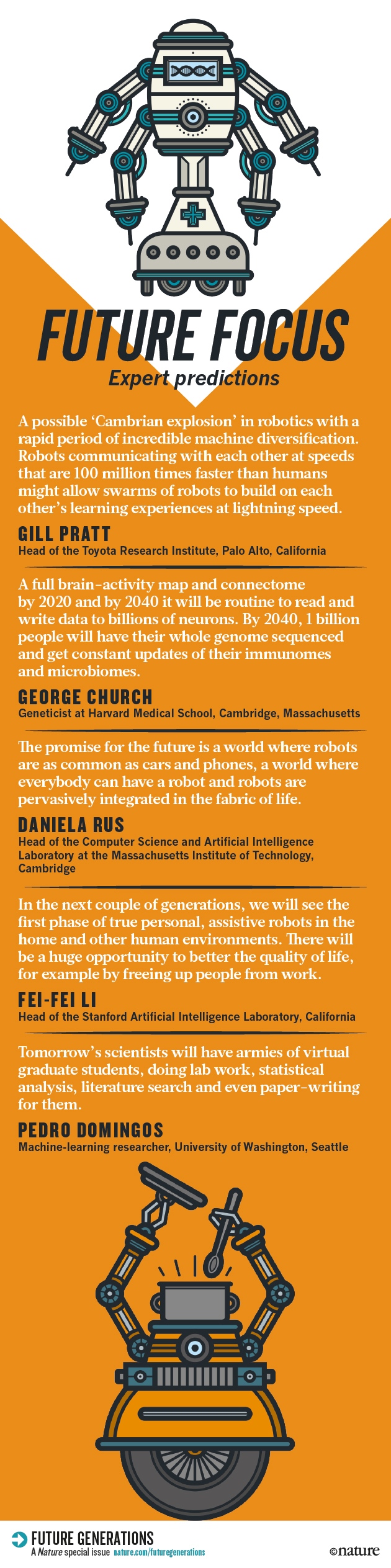
Computer science: The learning machines
Source: https://www.nature.com/news/computer-science-the-learning-machines-1.14481
Facial Recognition
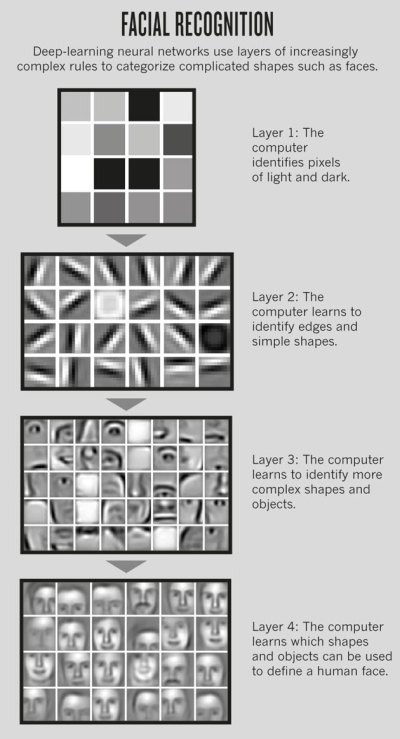